Statistics, in particular, plays a vital role in data science and can come in handy across industry verticals and data science job roles. The simplest definition of statistics is “data plus inference,” so to become a successful data science professional; one needs to have strong foundations in statistics.
Like it or not, statistics is an essential part of modern data science. At the same time, it’s also one of the most dreaded fields by many data science beginners, and this leaves a lot of newcomers wondering which books are the best to start with.
Worry not. This article provides a roundup of the top introductory book recommendations for data science students and professionals.
But before that, let us see what statistics for data science is?
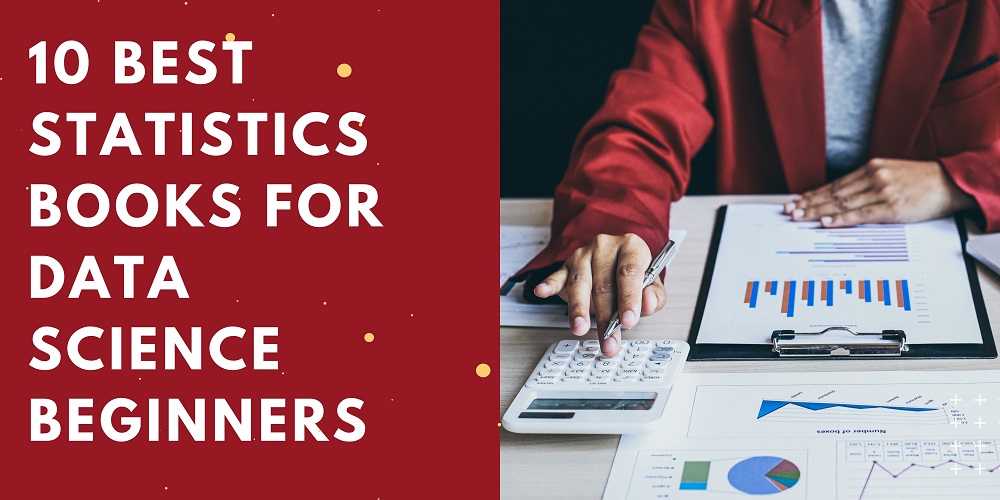
What is statistics for data science, and how is it used?
Statistics is all about applying data from multiple sources to form conclusions and make predictions. Statistics are used by data scientists to collect, assess, analyze, and derive conclusions from data and apply quantifiable mathematical models to relevant variables.
Despite statistics’ lack of popularity among data scientists in the past, it plays a crucial role in improving data analysis, prediction, and inference. It assists in filtering through data and simply presenting the results, thereby discovering hidden patterns, which is essential for data-driven decisions.
If you want to expand your knowledge of statistics for data science and understand how it is used in real-world applications, a data science course in Bangalore can be the right place for you.
What are the Best Statistics books for data scientists?
Statistics books are ideal for helping beginners learn fundamental statistical methods that form the foundation of today’s data science.
The books mentioned here are based on critical factors, including recommendations by statisticians, difficulty level, readability, and content.
1. Naked Statistics: Stripping the dread from the data
by Charles Wheelan
This book is written in a highly realistic manner that brings statistics to life. The book is filled with amazing concepts presented in an exceptionally unique style that makes statistics simple to study and embrace. This is the best statistics book for data science that explains everything from basic concepts like normal distribution to complex methods for data analysis algorithms.
2. How to lie with statistics
by Peter Bruce and Andrew Bruce
This is an excellent book for mastering the basics of statistics for beginners. The author explains principles like correlation, regression, and inference clearly and concisely. He discusses further how carelessness can affect data and how statistical graphs can be used to determine the truth. The book is relatively old and outdated, but its concepts are still applicable in the modern world.
3. Head-First statistics – A brain-friendly guide
by Dawn Griffiths
This well-known book presents information in a narrative and storytelling format. Each topic is explained using real-world examples to facilitate the learning process. This is the best option if you wish to strengthen your understanding of statistical fundamentals.
This book focuses on:
- Descriptive statistics ( mean, mode, median, etc.)
- Inferential statistics (correlation, hypothesis testing, etc.)
- Probability distributions ( binomial distribution, normal distribution, and Poisson distribution, among others.)
4. Practical statistics for data scientists
by Peter Bruce and Andrew Bruce
Practical statistics for data scientists is for data scientists who are familiar with the R programming language and want to learn how to apply statistical approaches while avoiding their misuse. The topics discussed in this book include data structures, datasets, regression, probability, statistical experiments, and machine learning. The source code is accessible in Python and R.
5. Introduction to statistical learning
by Gareth M. James, Daniela Witten
Introduction to statistical learning is a book for practicing data scientists. The primary focus is on bridging the gap between statistics and machine learning. As a result, you’ll be familiar with all of the most popular supervised and unsupervised machine learning algorithms. Since the practical elements of algorithms have been illustrated using R, R users will have an advantage. In addition to theory, this book explores the use of machine learning algorithms in real-world applications.
6. Think Stats
by Allen B. Downey
This book is perfect for those just starting out on their journey to learn statistics.
Think Stats introduces probability and statistics to Python programmers and focuses mainly on data science-related topics. This book is also geared toward experienced programmers with Python code examples, teaching them statistical principles through real-world data analysis examples and encouraging them to work with real datasets. It covers statistical reasoning, correlation, hypothesis testing, distributions, and analytical procedures.
Downey’s other book, Think Bayes, explores the use of Python programming to solve statistical problems.
7. Discovering Statistics Using R
by Andy Field, Jeremy Miles
This book is written in an easy-to-follow style and is designed to help one get started using R statistical software programs. It covers everything from basic concepts like probability theory to advanced topics like regression analysis and time series analysis. The author also includes plenty of exercises at the end of each chapter for learners to put what they’ve learned into practice.
8.Statistics in Plain English
by Timothy C. Urdan
This is the best introductory statistics book for data science beginners in many ways. It is a good resource for anyone who wants to learn about statistics but has no idea where to start. The author provides an overview of statistical concepts and how they’re used in real-world situations, using plain language and clear examples to explain each point. In each chapter, a statistical approach is described and illustrated with an example, including central tendency and characterizing distributions, t-tests, regression, repeated measurements, and factor analysis.
The book covers all of the essential topics that matter to data scientists:
- Descriptive statistics,
- Probability and probability distributions,
- Hypothesis testing,
- Regression analysis, and more.
There are also chapters on experimental design and linear models that are relevant to data science workflows.
9. The Art of Data Science
By Elizabeth Matsui, Roger. D Peng
Written by a professor at Columbia University, this book covers all the essential areas needed for a strong foundation in data science: programming skills (R), statistical thinking skills (including probability theory), machine learning techniques (including deep learning), as well as modeling approaches such as classification trees, decision trees, and Bayesian networks.
10. Pattern Classification
By Richard.O Duda, Peter E
Pattern Classification, a popular book that explains mathematical concepts and algorithms, was first published in 1973 and revised a few years ago. The book focuses on neural networks, machine learning, and statistical learning using classic and cutting-edge techniques. This book provides detailed explanations of methodologies and historical references through examples, case studies, and algorithms.
So, which ones are you adding to your bookshelf now?
Conclusion:
Whether you are new to the world of data analytics or an experienced professional simply looking to brush up on your knowledge, we hope this post helps you find the statistics books that meet your needs.
Data Science is fast becoming the buzzword for businesses. There are numerous training classes and data science courses available to understand better how statistics works and its relation to the actual data world. The above books offer some of the best descriptions, from beginner statistics concepts to data mining, Bayesian, probabilistic, and Information Theory ideas for all levels of age groups in a straightforward language.
AUTHOR?S BIO:
I am Sairaj Tamse, a data science enthusiast and passionate blogger who loves to write technical and educational contents like data science course, Machine learning and Artificial Intelligence. I always believe in smart learning processes that help people understand concepts better, and writing is my way of doing so. The only thing I like about people is how they value learning time, which motivates me to work more on them. I always prefer writings that will help tech enthusiasts in succeeding their careers.

As the editor of the blog, She curate insightful content that sparks curiosity and fosters learning. With a passion for storytelling and a keen eye for detail, she strive to bring diverse perspectives and engaging narratives to readers, ensuring every piece informs, inspires, and enriches.